Algorithmic Futures: Impact on Financial Markets
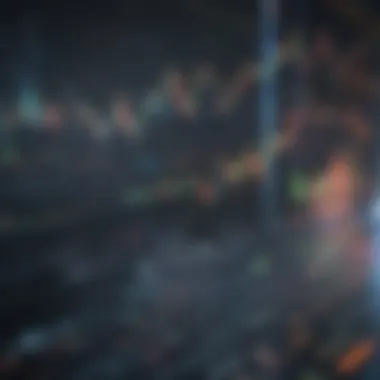
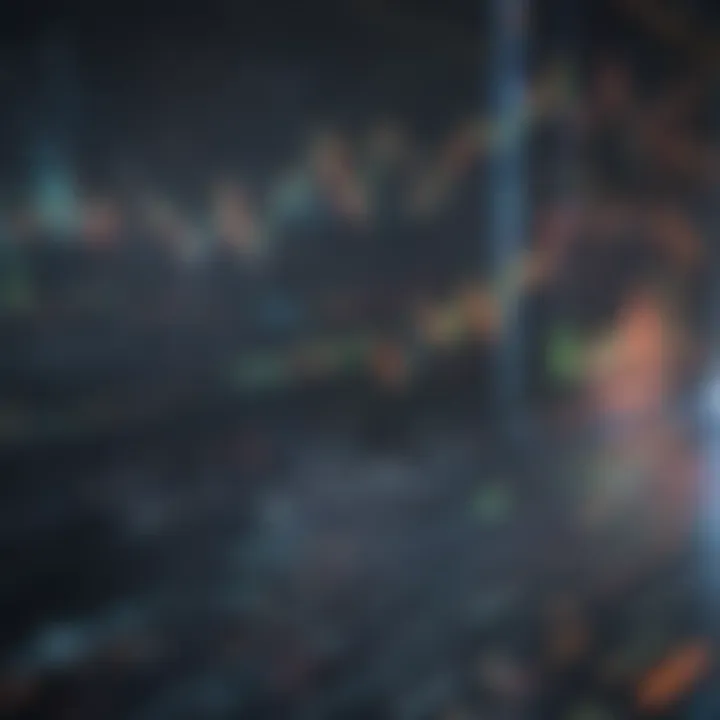
Intro
In the current financial landscape, algorithms have become the unsung heroes driving decision-making processes in market operations. Their influence extends far beyond number crunching and trade executions. They play a pivotal role in shaping investment strategies and risk assessments. This article aims to unravel the intricacies of algorithmic trading, dissect the accompanying ethical considerations, and highlight the implications of these computational techniques on individual investors and market economies as a whole.
Understanding algorithms is akin to peeling an onion; there are layers of complexity behind the shiny surface. The debate centers around whether this technology is a boon or a bane, and thatâs where many investors find themselves scratching their heads.
With the ongoing evolution of big data and artificial intelligence, financial professionals have to navigate through new paradigmsâwhere speed is king and accuracy reigns supreme. Letâs delve deeper into this captivating domain and start by defining the essential terminology that will guide our exploration.
Prolusion to Algorithmic Futures
The landscape of finance has transformed dramatically over the last few decades, a change that has been propelled, in large part, by the advent of algorithmic trading. Understanding this transformation is essential, as it illustrates how algorithms are not merely tools but have become pivotal players in the financial arena.
Algorithmic futures can be seen as a lens through which to view the evolving dynamics of investment strategies. This evolution is crucial for investors, financial advisors, and analysts, who must navigate this complex environment. The focus here is not solely on the mechanics of trading but also on the implications these algorithms carry for decision-making and economic consequence.
Definition of Algorithmic Futures
At its core, algorithmic futures encompasses the advanced technology and methods used to automate trading processes in financial markets. These systems leverage mathematical models and sophisticated computational techniques that allow for rapid execution of trades based on pre-defined criteria. In practical terms, one might say itâs like having a highly efficient assistant whirling around the stock exchanges, making calculated trades at lightning speed, far quicker than any human could manage.
Understanding what algorithmic futures are lays the groundwork for delving into their historical emergence, mechanisms, and relevance in todayâs ever-evolving financial landscape.
Historical Context
The introduction of algorithmic trading dates back to the 1970s, coinciding with the rise of technological advancements. Initially, the emphasis was on automated order execution, but the scope quickly broadened. By the 1980s, innovations like the Thomson Reuters Quotron paved the way for extensive data processing that would later allow for the complex algorithms we see today.
Fast-forward to the dot-com boom of the late 1990s, the fusion of technology and finance became evident, quickly catching the attention of institutional investors. By the early 21st century, algorithmic trading had become mainstream. The infamous flash crash of 2010, where the Dow Jones Industrial Average dropped 1,000 points in mere minutes, underlined both the power and risks inherent in these systems. This incident raised alarms about market stability and constructed a narrative that still influences regulations today.
Relevance to Modern Finance
Today, algorithmic trading doesnât exist in a vacuum; it resonates deeply within the fabric of modern finance. Many institutional investors rely on sophisticated algorithmic strategies to manage their portfolios, aiming for improved efficiency and reduced transaction costs.
Moreover, the proliferation of big data has allowed traders to concoct more complex trading strategies, blending speed with accuracy. Algorithms sift through vast datasets, identifying patterns and executing trades based on real-time information.
"In the realm of finance, speed can be the difference between profit and loss. Algorithmic futures epitomizes this crucial fact."
As financial markets become increasingly interconnected, the role of algorithmic trading is set to expand further. Understanding its relevance offers valuable insights into current investment landscapes and can aid in predicting future market movements. Investors and financial professionals who appreciate these aspects will find themselves better equipped to navigate this rapidly changing environment.
In summary, delving into the concept of algorithmic futures not only highlights its significance in trading but also reveals its overarching impact on market dynamics and financial stability.
The Mechanics of Algorithmic Trading
Understanding the mechanics of algorithmic trading is essential for anyone looking to navigate the complexities of modern financial markets. This section illuminates how these algorithms operate, their various types, and the implications they have on trading strategies. A firm grip on these mechanics isnât just beneficial; itâs pivotal for investors, financial advisors, and analysts. As financial landscapes evolve, discerning these underlying processes equips stakeholders with the necessary insight to make informed decisions.
How Algorithmic Trading Works
At its core, algorithmic trading refers to the use of complex mathematical models and formulas to execute trades at speeds and volumes that are impossible for human traders to achieve. It often involves the automation of trading processes, utilizing algorithms to identify trading opportunities based on algorithms designed for specific conditions.
In a practical sense, algorithmic trading involves gathering data from various sources, analyzing it in real time, and executing trades based on predetermined criteria. These algorithms analyze vast amounts of market data to forecast price movements, assess risks, and ultimately make decisions for trade execution.
Key components include:
- Market Data Integration: Continuous monitoring of market changes to inform trading decisions.
- Order Execution Algorithms: Determining how to execute trades efficiently without disrupting the market price.
- Risk Management Features: Built-in protocols that help in minimizing losses by setting stop-loss orders and managing position sizes.
Types of Trading Algorithms
Trading can be categorized based on the underlying strategies employed by different algorithms. Understanding these types is crucial as they significantly shape market behavior and investor strategies.
High-Frequency Trading
High-frequency trading (HFT) exemplifies a cutting-edge method that employs sophisticated algorithms to initiate trades at lightning speeds, often within fractions of a second. The key characteristic of HFT is its pace; these algorithms analyze multiple markets and execute orders based on the results almost instantaneously. The benefit of HFT lies in its ability to capitalize on minute price discrepancies that occur almost fleetingly, making it an attractive strategy for those who thrive in fast-paced environments.
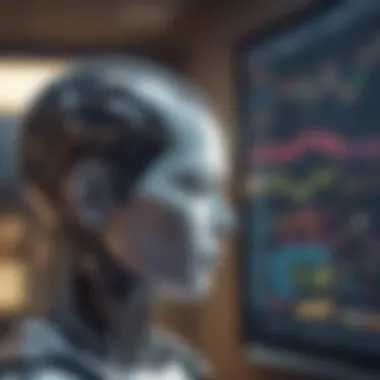
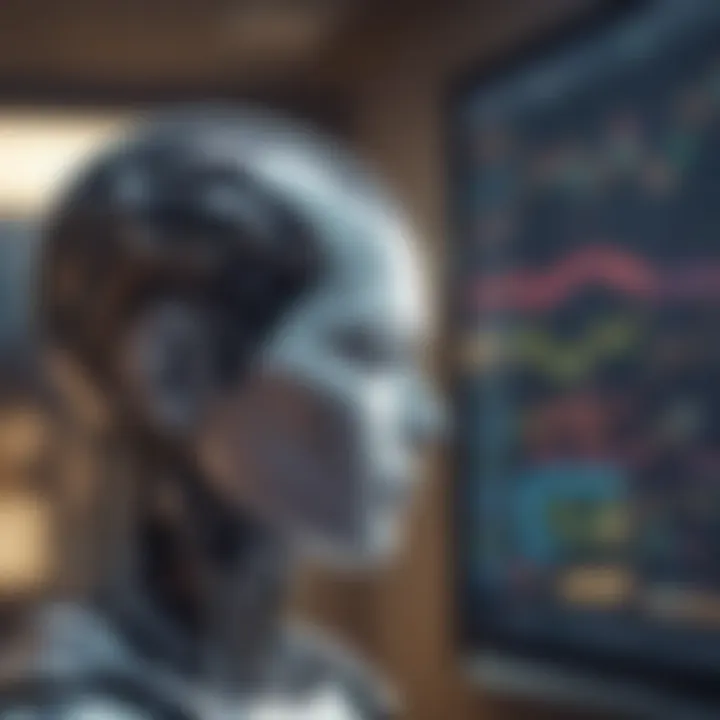
An advantage of HFT is liquidity provision, as traders frequently enter and exit positions, fostering a more dynamic market. However, it also presents disadvantages; slippage can occur due to market volatility, leading to losses, and high-frequency firms may bear significant operational costs associated with prowess in technology and algorithm development.
Statistical Arbitrage
Statistical arbitrage leverages mathematical models to identify trading opportunities based on historical price data. The primary strength of this strategy lies in its dependence on statistical probabilities rather than mere market sentiment; this is crucial for isolating trades that could yield profit over time.
What makes statistical arbitrage particularly appealing is its reliance on pairs of correlated securities â often in a rotating fashion â which allows systematic traders to explore the price divergences and converge them back to the mean price. Its advantage lies in its rigorous data analysis approach, which often leads to greater predictability. Yet this method isnât without its drawbacks; it requires extensive backtesting and adjustments, which could inadvertently miss out on real-time opportunities.
Market Makers
Market makers play a critical role in ensuring liquidity within financial markets by maintaining inventory for various assets and continuously quoting buy and sell prices. They help stabilize prices by being ready to buy when others want to sell, and vice versa. The key characteristic of market making lies in their ability to facilitate trades; they are effectively the intermediaries between buyers and sellers.
One of the benefits of market making is that it often leads to reduced bid-ask spreads, enhancing overall market liquidity. However, risks abound; market makers can suffer significant losses in volatile conditions where prices swing dramatically, leading to instances of illiquidity.
To sum up, understanding the mechanics behind algorithmic trading and the various types of algorithms is pivotal. The intricacies of each method yield unique opportunities and challenges that practitioners need to navigate with finesse to optimize their trading performance.
Impacts on Market Dynamics
Understanding the impacts on market dynamics as driven by algorithmic trading is paramount in grasping how financial ecosystems evolve in the face of technological advancements. Algorithmic trading reshapes traditional market paradigms, enhancing efficiency while simultaneously introducing new complexities. This dual-edge aspect encompasses various factors that merit examination.
Volatility and Market Efficiency
Market volatility, often perceived as a double-edged sword, can lead to both gains and losses in the investment landscape. With the rise of algorithmic trading, the marketplace often sees increased volatility. Algorithms can process vast amounts of data and execute trades at breakneck speeds, sometimes exacerbating price fluctuations. This phenomenon happens particularly during periods of market stress when algorithms are programmed to react instantly to negative news or economic indicators.
However, itâs essential to recognize that this same algorithmic approach also contributes to enhanced market efficiency. Lower transaction costs, narrower bid-ask spreads, and quicker responses to market changes can lead to a more dynamic trading environment. Financial institutions leveraging algorithmic strategies can operate with a level of precision previously unattainable, allowing them to absorb some of this volatility while profiting from it.
"The efficiency of markets often hinges on complexity that algorithmic trading must navigate, creating both opportunities and risks."
Furthermore, the behavior of high-frequency traders can signal potential volatility. Their tactics often involve arbitrage opportunities that, when taken rapidly across numerous platforms, can provide liquidity in turbulent times. As they engage in rapid buy and sell cycles, this can lead to short-term spikes in volatility, influencing broader market sentiments.
Role in Price Discovery
The process of price discoveryâthe mechanism through which market prices adjust based on supply and demandâhas been markedly influenced by algorithmic trading. Traditionally, price discovery relied heavily on human judgment, which, though often sound, was limited by cognitive biases and slower reaction times. With algorithms, price adjustments occur almost in real-time, incorporating new information much faster.
This efficiency in price discovery holds several advantages:
- Rapid Response to Information: Algorithms can assimilate information from diverse sources instantaneously. This allows for swift adjustments to prices, reducing the frictions often associated with delayed reactions.
- Elimination of Human Error: Human traders, in their decision-making processes, can inadvertently introduce biases or errors. In contrast, automated trading systems operate on a pre-defined set of rules, minimizing these uncontrollable variables.
- Increased Market Depth: The blend of high-frequency trading and algorithmic utilization often leads to deeper markets, where more buy and sell orders are instantly available. This depth can create more stable pricing structures, albeit also potentially leading to sudden gaps in pricing if thereâs a significant enough shift in market sentiment.
Understanding these impacts on market dynamics helps investors, financial advisors, and analysts navigate the intricate landscape of algorithmic trading. Recognizing how volatility interplays with aggression in price discovery aids in strategic decision-making. The algorithms have not only transformed how trades are executed but also how value is perceived and established within the marketplace.
Risk Management in Algorithmic Trading
When we talk about algorithmic trading, one cannot overlook the vital aspect of risk management. This is, quite frankly, the backbone of any trading strategy, especially when dealing with high-speed algorithms and constantly shifting market conditions. Without effective risk management, traders might as well be playing darts blindfolded; the chance of hitting a bullseye shrinks considerably. The complexities of the financial landscape necessitate rigorous risk management practices to shield investments from potential calamities.
Risk management in this context involves identifying possible pitfalls, quantifying them, and putting in place measures to avoid or minimize these risks. Algorithms can turn a seemingly bad trading day into devastation if not properly monitored. A robust risk management strategy helps in setting clear boundaries for loss exposure, outlining how much capital can be risked on a single trade, known as position sizing, and establishing hard stop-loss levels to mitigate losses when things go south.
The beauty of algorithmic trading lies in its efficiency. With algorithms running at lightning speed and executing trades based on predefined criteria, one must ensure these parameters include strict risk controls. This dual focus on agility and caution is what allows traders to navigate the unpredictable waters of financial markets.
In essence, effective risk management enables a trader to safeguard their investments, while still capitalizing on potential gains from well-thought-out strategies. It is not merely enough to chase profits; the focus should also embrace preserving capital, thus allowing for sustained participation in the market.
Identifying and Mitigating Risks
Now, let's get into the nuts and bolts of identifying and mitigating risks. In algorithmic trading, risks come in various flavors â market risk, liquidity risk, technology risk, and even operational risk, to name a few. Each of these presents a different set of challenges that require targeted strategies to manage.
- Market Risk: This is the heavy hitter. It refers to the potential losses that could arise from fluctuations in market prices. Algorithms are sensitive to these changes, thus requiring continuous monitoring and adjustments.
- Liquidity Risk: Imagine you have a boat-load of shares to sell, but there are no buyers in sight. Thatâs liquidity risk â the risk of not being able to buy or sell assets quickly enough without causing a significant impact on their price. Algorithms need to factor in current market conditions and volume.
- Technology Risk: In a world governed by tech, things can and often do go haywire. System failures, data breaches, and incorrect coding create vulnerabilities that can lead to major financial setbacks. Continuous testing and updates of the system are essential.
- Operational Risk: This encompasses the daily activities of running an algorithm, including human error and process failures. Proper training and protocols can help mitigate this.
To narrow down on these risks, here are a few strategies traders can leverage:
- Diversification: Don't put all your eggs in one basket. Spread your investments across various assets.
- Regular Monitoring: Keep a keen eye on market movements and adjust strategies as needed.
- Risk Assessment Tools: Use sophisticated software and tools to measure risk potential.
- Stress Testing: Simulate extreme market conditions to see how your algorithm performs.
By identifying these various risks upfront and implementing measures to combat them, traders can stay one step ahead and avoid the pitfall of unexpected losses.
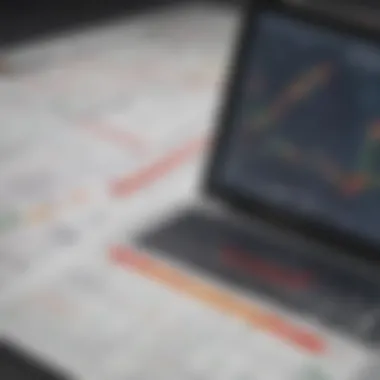
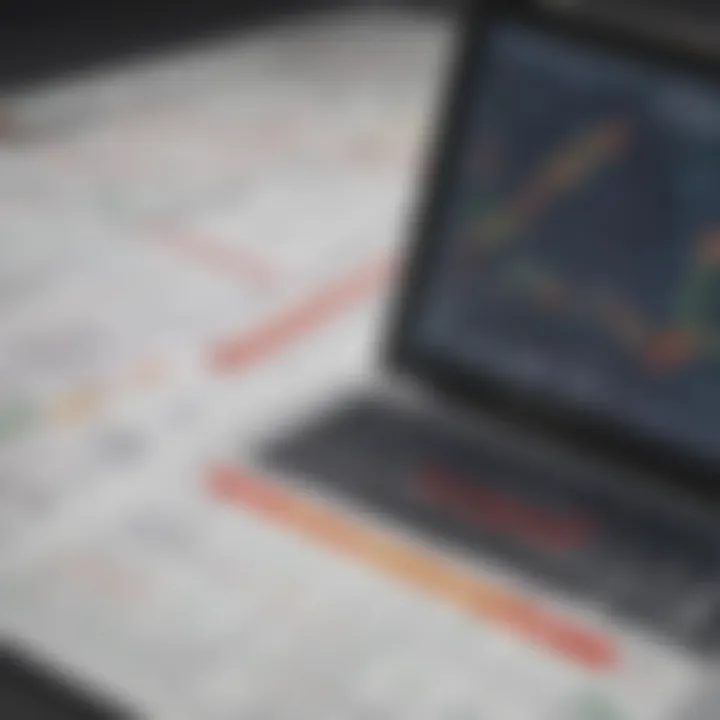
The Role of Backtesting
Backtesting is like having a time machine for your trading strategies. It allows you to look back at historical data and test how your algorithms would have performed under various market conditions. Think of it as a rehearsal before the big performance â crucial for fine-tuning your strategy to get the best results when the curtain rises.
Backtesting provides insights and metrics that reveal the efficacy of an algorithmic strategy. You can evaluate the profit-and-loss ratio, drawdowns, win rate, and risk-reward ratios without risking real capital. This is how traders uncover flaws in their algorithms and make adjustments accordingly.
- Performance Metrics: Essential performance indicators can be generated during the backtesting phase. You can spot trends that could influence trading decision-making moving forward.
- Historical Analysis: Analyzing how market environments influenced algorithm performance allows traders to better understand potential future behaviors.
- Refining Strategies: Based on backtesting results, traders can refine their algorithms. This could involve optimizing parameters or modifying entry and exit strategies.
However, itâs important to treat backtesting with caution. Just because an algorithm performed well in the past doesn't guarantee future success. Markets change, and historical performance might not be indicative of future outcomes. Yet, when paired with sound risk management practices, backtesting becomes an indispensable tool in a traderâs arsenal, mitigating potential losses while crafting a path toward growth.
Ethical Considerations in Algorithmic Finance
Algorithmic finance operates in a complex world where technology marries economics to create powerful tools for trading and investment. However, as these algorithms dictate a significant portion of market activities, ethical considerations come to the forefront. The potential for unintended consequencesâranging from market manipulation to societal impactsâdemands a rigorous examination of the ethical compass guiding these financial technologies. By understanding these ethical implications, investors and analysts can navigate the murky waters of algorithm-driven markets more effectively.
Market Manipulation Concerns
Market manipulation is a major concern in algorithmic trading. The sophisticated techniques used by high-frequency traders and others create scenarios ripe for exploitation. For instance, some algorithms can engage in practices like quote stuffingârapidly placing and then canceling orders to confuse market participants, leading to erroneous price shifts. This form of manipulation not only distorts market efficiency, but also erodes trust among long-term investors.
- Types of Manipulation:
- Spoofing: Placing orders with no intention to execute, only to cancel them before fulfilling, affecting prices.
- Wash Trading: Buying and selling the same asset rapidly to create misleading volume metrics.
Consequently, market manipulation can lead to increased volatility, where unsuspecting investors might make poor decisions based on artificially inflated prices. Addressing these risks is crucial, not only for maintaining market fairness but also for preserving the integrity of financial institutions.
Transparency and Accountability
As algorithmic trading grows, establishing transparency and accountability becomes critical. Investors need to know how algorithms make decisions, especially when large-scale trading impacts stock prices. Financial firms often treat their algorithmic strategies as trade secrets, leaving a veil of opacity. This situation raises questions:
- Who is responsible? In cases of market failure, it can be difficult to pinpoint who is liable if an algorithm acts against the interests of fairness.
- How is data used? If data used in algorithms isn't publicly accountable or verified, unethical practices might seep into how trades are executed.
Transparency can build trust between investors and firms, ensuring that algorithms benefit rather than exploit. For example, public disclosures of trading parameters and regular audits could serve as measures to hold financial firms accountable, thereby minimizing unethical practices. To foster a healthy trading environment, it is vital that ethical standards are rigorously enforced to hinder manipulation and advocate for transparency in algorithmic finance.
The unregulated domain of algorithmic finance pushes critical boundaries, calling for greater oversight and ethical reflection.
The Role of Big Data in Algorithmic Futures
Big data has emerged as a cornerstone in the realm of financial modeling and trading strategies. Its role cannot be understatedâit's not merely about numbers, but rather the insights derived from voluminous datasets that create opportunities for algorithmic decision-making. With staggering amounts of data generated every second, the financial sector has begun to tap into this wealth of information to gain competitive advantages and improve investment outcomes.
The intersection of big data and financial trading manifests in various facets. Primarily, it enhances predictive models that guide traders and analysts in their decision-making processes. This section will explore in depth how data collection practices and the relevant privacy and security concerns shape the future of algorithmic trading.
Data Collection Practices
Data collection in financial markets is akin to digging for gold in a mine. Investors and institutions employ multifarious methods to gather data, from traditional market feeds to innovative sources such as social media sentiment analysis and IoT devices. Here are some prominent practices:
- Market Data Feeds: Professional traders often subscribe to market data feeds which supply near real-time data on stock prices, trading volumes, and other vital statistics. This data is the lifeblood of algorithmic trading systems, allowing them to react swiftly to market changes.
- Alternative Data Sources: Nowadays, successful investors also look beyond traditional data sources. By analyzing satellite images of retail store parking lots, for instance, traders can gauge foot traffic to forecast company performance even before quarterly results are announced. This kind of alternative data is becoming crucial for trading strategies.
- Natural Language Processing: Financial news outlets and social media can generate insights into market sentiment. Algorithms utilize natural language processing to analyze articles and tweets about companies, allowing traders to gauge public sentiment and potential impact on stock performance.
Collecting data is not just about quantity; quality is paramount as well. The efficacy of trading algorithms hinges on the relevance and accuracy of the data they process. Unreliable data can lead to misinformed trading strategies, so institutions invest heavily in data validation methodologies to ensure integrity in their datasets.
Data Privacy and Security Issues
While the importance of data is clear, it brings forth critical questions surrounding privacy and security. These issues heighten as financial entities navigate the complexities of handling sensitive data. Here are key concerns:
- Regulations and Compliance: Compliance with data protection regulations, such as the General Data Protection Regulation (GDPR) in the European Union, poses challenges for data collectors. Violations can lead to heavy fines and reputational damage, prompting companies to rethink their data collection and storage strategies.
- Data Breaches Risks: With great data comes great responsibility. Financial institutions are prime targets for cyberattacks, leading to the risk of sensitive information falling into the hands of malicious actors. Investing in robust cybersecurity measures is essential for maintaining client trust and safeguarding personal data.
- Ethical Data Use: The fine line between data utilization and invasion of privacy is often blurred. Companies must not only be cautious about how they collect data but also how they utilize it. Ethical considerations are paramount, as algorithms learn from past behaviors, which can inadvertently lead to biased decision-making processes.
Artificial Intelligence and Machine Learning in Finance
The significance of Artificial Intelligence (AI) and Machine Learning (ML) within the context of finance cannot be understated. These technologies are revolutionizing how transactions are executed, investments are managed, and risks are assessed. In an industry where split seconds can mean substantial gains or losses, the ability to process vast quantities of data efficiently and effectively is crucial.
AI and ML empower financial institutions to not only enhance their trading strategies but also to tailor them in line with changing market dynamics. Investors increasingly rely on complex algorithms to sift through numerous variables, identifying patterns and predicting outcomes, thereby maximizing their profit potential. The ability of these technologies to learn from historical data adds a layer of predictive capability that manual analysis simply cannot match.
The benefits extend beyond just speed and efficiency. With advanced algorithms, financial models are becoming more audacious. For instance, consider a scenario where a hedge fund utilizes AI to shift allocations in real-time based on predictive analytics rather than traditional methods. This shift not only enhances competitive advantage but also allows for a better response to market volatility.
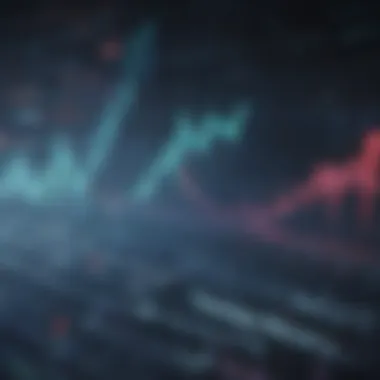
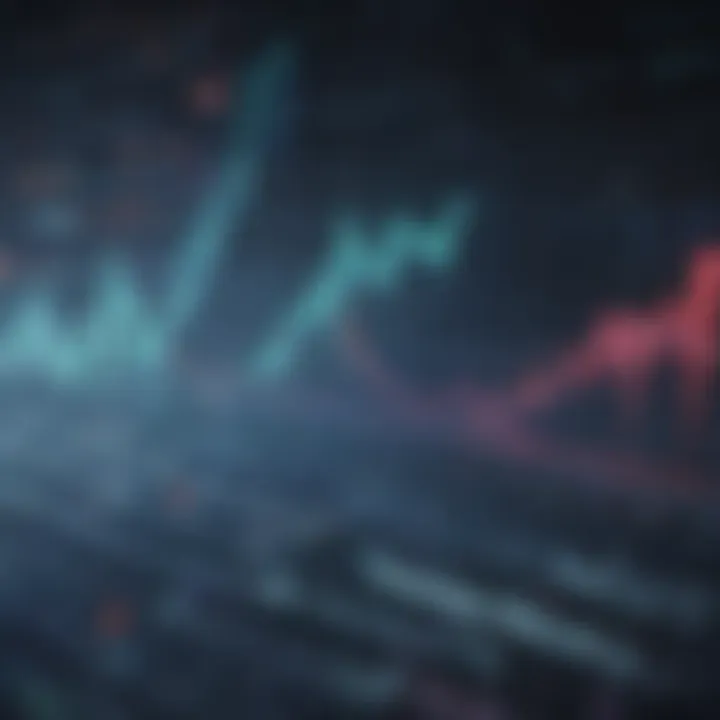
Moreover, AI systems can refine their strategies by continuously learning from new data inputs. This is akin to teaching a dog new tricksâonce it figures something out, it might even discover better, more efficient methods along the way. Therefore, the integration of AI and ML in trading strategies is not just a trend; itâs becoming a cornerstone of modern finance.
Integration of AI in Trading Strategies
Integrating AI within trading strategies marks a pivotal evolution in finance. Algorithms are designed to analyze vast pools of data, often at speeds that would bewilder a human trader. This could involve monitoring real-time market trends, social media sentiment, and economic news eventsâall of which can influence stock prices.
Several prominent trading firms employ AI-driven strategies such as:
- Sentiment Analysis: Algorithms gauge public sentiment towards certain stocks based on social media activity and news coverage, predicting potential price movements.
- Algorithmic Arbitrage: By using AI to identify pricing discrepancies across different markets, traders can execute buy/sell orders almost instantaneously.
- Predictive Modeling: Algorithms utilize past price movements and trading volumes to forecast future behavior, offering insights that are often spot on.
"The future of finance is not just about numbers; itâs about a dance of algorithms and data, producing rhythms that can guide investments successfully."
These methods enable practitioners to systematically exploit opportunities that humans may overlook due to limitations in processing speed and data analysis. As this technology improves, so too do the complexities of the strategies that underpin successful trading.
Bias and Ethical Dilemmas
While AI offers tremendous advantages, it is essential to acknowledge the ethical dilemmas it presents. Bias in algorithms is a significant issue that can lead to skewed results. For example, if historical data reflects past prejudices or systematic inequalities, the AI trained on this data could propagate these biases in its trading strategies. This may not only lead to moral concerns but can also result in biased financial decisions that could have sizeable impacts on markets.
Several considerations regarding ethics and bias include:
- Data Integrity: Ensuring that the data is collected and used responsibly is paramount. Data sets must be as representative and balanced as possible to mitigate embedded biases.
- Transparency: There is often a black box surrounding how AI systems make decisions. The lack of insight into these processes can lead to mistrust among investors.
- Regulation: As algorithms have an increasing role in financial markets, regulatory bodies may need to enforce guidelines ensuring AI usage aligns with ethical standards.
These issues create an imperative for financial entities to not only innovate but also reflect upon the principles underlining their strategies.
In summary, while AI and ML herald a new era for financial strategies, the balance between leveraging technology for advancement and maintaining ethical integrity remains a critical focal point.
Future Trends in Algorithmic Trading
The landscape of algorithmic trading continues to shift beneath our feet, shaped by technological advancements and regulatory changes. Understanding these trends isn't just beneficial; it's crucial for those involved in finance today. This section delves into the emerging technologies that are reshaping trading strategies, and how regulation is adapting to these changes. By the end, readers should grasp the dynamic nature of the sector and the implications for individual and institutional investors alike.
Emerging Technologies
In recent years, the rise of machine learning and artificial intelligence has redefined algorithmic trading. What was once reserved for complex statistical models and high-speed transactions is now evolving into systems capable of learning and adapting.
- Machine Learning Models: Traders now use machine learning models that can analyze vast amounts of data in real time, uncovering patterns that might elude the human eye. This leads to more informed trading decisions.
- Natural Language Processing (NLP): Algorithms are now being developed to interpret news articles, social media feeds, and financial reports. By utilizing NLP, traders can gauge market sentiment and make trades based on news that hasnât yet crossed the general market consciousness.
- Blockchain Technology: The impact of blockchain extends well beyond cryptocurrencies. It offers transparent, tamper-proof transaction records which can enhance the efficiency of settling trades and reduce fraud.
"In a world increasingly governed by data, those who harness the power of algorithms will dominate the financial landscape."
Moreover, advancements in hardware, particularly in the realm of quantum computing, promise to exponentially increase computational power. This will allow traders to run more complex models and simulations, granting a competitive edge in the fast-paced world of finance.
Evolution of Regulation
As algorithmic trading becomes more prevalent, the frameworks governing these practices are undergoing transformations to catch up with the pace of innovation. Regulatory bodies are rethinking their strategies to mitigate risks while encouraging innovation.
- Increased Scrutiny: Following various market disruptions attributed to algorithms, regulators are more vigilant. They are introducing stricter guidelines on the use of algorithms to shield the markets from high-frequency trading's potential volatility.
- Transparency Requirements: Thereâs growing demand for transparency in trading algorithms. Regulatory agencies may require firms to disclose codes or strategies to ensure equitable play in the markets.
- Testing and Compliance: Firms employing algorithmic trading must now undergo rigorous testing procedures to ensure that their systems behave as intended in various market conditions. This includes stress-testing under different scenarios to detect potential failures before they occur.
- International Coordination: Given that trading often occurs across borders, global collaboration among regulatory bodies is becoming essential. Standardizing regulations could help manage risks that arise from different jurisdictions' policies.
The End: Navigating Algorithmic Futures
In a swiftly evolving financial landscape, the concept of algorithmic futures stands out as a pivotal element shaping market interactions and investment practices. As we draw this article to a close, itâs essential to reflect on the profound implications that algorithmic trading has for individual investors and the overall market structure. The future promises not only opportunities but also challenges that require a keen understanding of the intricacies involved.
Implications for Individual Investors
Individual investors, whether seasoned or novices, must grasp the reality that algorithmic trading changes the rules of engagement in financial markets. Here are some key implications to note:
- Access to Advanced Tools: With algorithmic trading gaining traction, many retail investors can now access sophisticated trading software and strategies that were once exclusive to institutional players. Retail platforms are increasingly providing tools that allow individuals to implement algorithmic strategies.
- Informed Decision-Making: Equipped with data-driven insights and real-time analytics, individual investors are better positioned to make informed decisions. However, while algorithms can process vast amounts of information rapidly, there remains the danger of over-reliance on them, potentially leading to impulsive trading behavior.
- Increased Competition: As more investors embrace algorithmic trading, the competitive landscape becomes fiercer. Savvy algorithms can quickly exploit market inefficiencies, making it harder for individual investors to compete unless they are well-versed in algorithmic strategies.
- Heightened Awareness of Risks: Understanding the underlying risks associated with algorithmic trading is crucial. From technical glitches to market anomalies, individual investors need to be aware that algorithms are not infallible. Hence, a solid grasp of risk management practices is indispensable.
The stakes are high and the learning curve steep, hence individual investors may want to educate themselves on not only how to utilize these technologies but also the broader implications on their investment approach.
Looking Ahead
As we cast our gaze toward the horizon of algorithmic futures, several trends unfold that merit attention:
- Advancement in Technology: Emerging technologies such as machine learning and AI are likely to continue enhancing algorithms, resulting in ever more sophisticated trading strategies. Investors must stay abreast of these developments to adopt beneficial practices and avoid falling behind.
- Regulatory Evolution: As algorithmic trading increases, regulatory bodies will respond by tightening controls and implementing new frameworks designed to address potential market abuses. Investors need to stay informed regarding changes in regulations that could affect their strategies.
- Integration of Ethical Standards: The conversation around ethical considerations remains pertinent. As algorithmic trading continues to blur the lines between fair trading practices and manipulation, a dialogue around accountability will be vital in shaping a responsible trading environment.
- Focus on Sustainability: Investors may increasingly pursue algorithms designed with an emphasis on sustainable investment strategies. This shift could redefine market priorities toward ethical investing and responsible asset management.
For more information on algorithmic trading dynamics, you can visit Wikipedia or engage with discussions on platforms like Reddit that dive into real-world experiences and strategies.
This closing section provides a comprehensive synthesis of the information presented while offering actionable insights and considerations for those invested in the world of algorithmic trading.