In-Depth Exploration of Wall Street Financial Modelling
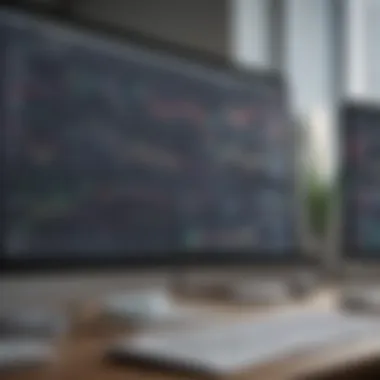
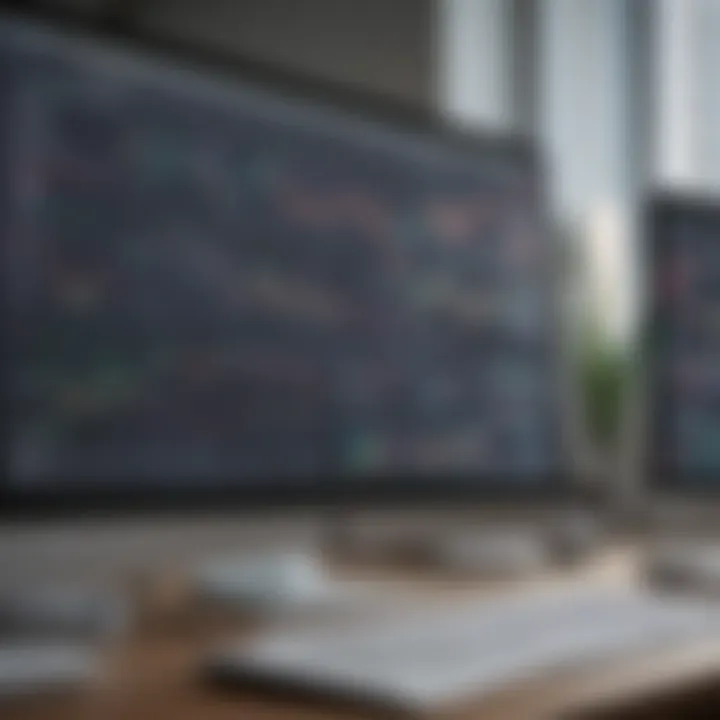
Intro
Financial modelling is a critical component of Wall Street's operations. It is the backbone that supports investment decisions, company valuations, and strategic planning across the finance industry. As complex as it may sound, understanding the essentials of financial modelling can empower investors, analysts, and advisors alike to make informed choices in a fast-paced environment.
In this piece, we'll embark on a journey through the ins and outs of financial modelling on Wall Street. We will demystify key terminologies, examine various methodologies, and underscore the influence of technology on modelling practices. Our goal is to equip you with insights that are not only theoretical but practical—essential for enhancing your decision-making prowess when navigating financial landscapes.
Ready? Let’s dive in.
Investment Dictionary
Understanding financial modelling is akin to knowing the lingo of a new environment. In this section, let’s go over some essential terms and definitions that are instrumental for anyone who wants to get a solid grip on Wall Street's secret language.
Key Terms and Definitions
- ** Discounted Cash Flow (DCF)**: A valuation method used to estimate the value of an investment based on its expected future cash flows. The cash flows are adjusted to reflect their present value to account for the time value of money.
- Beta: A measure that indicates the volatility of an asset or portfolio in relation to the overall market. A beta greater than one means that the asset is more volatile than the market, while a beta less than one indicates less volatility.
- Capital Asset Pricing Model (CAPM): A framework used to determine the expected return of an asset based on its systematic risk, essentially bridging the risk-return relationship in investments.
- Net Present Value (NPV): A method used to assess the profitability of an investment by calculating the difference between the present value of cash inflows and outflows over a given period.
Understanding Investment Jargon
Investment jargon often seems like a foreign language, laden with abbreviations and complex phrases. Here's a chance to break it down effectively:
- Asset Allocation: Refers to the process of dividing investments among different asset categories, like stocks, bonds, and cash. This strategy helps in diversifying risk.
- Liquidity: The ease with which an asset can be converted into cash. High liquidity means you can sell without a significant impact on the price.
- Leverage: Using borrowed capital to increase the potential return of an investment. While it can amplify gains, it can also magnify losses.
By familiarizing yourself with these terms, you lay the groundwork for mastering financial modelling techniques and their applications.
Financial Product Comparisons
With an understanding of the language, the next step is navigating through various financial products available in the market. Knowing the differences and similarities can steer you towards making more informed choices.
Side-by-Side Analysis of Popular Options
When applying financial modelling, comparing financial products side-by-side can be illuminating:
- Stocks vs. Bonds
- Mutual Funds vs. ETFs
- Stocks: Represent ownership in a company and can offer high returns but come with greater risk.
- Bonds: Typically considered safer, as they offer fixed interest payments; however, they generally yield lower returns compared to stocks.
- Mutual Funds: Managed by professionals, they pool money from many investors to purchase a diversified portfolio of stocks and/or bonds.
- ETFs (Exchange-Traded Funds): Similar to mutual funds but traded on stock exchanges, providing flexibility and potentially lower fees.
Selecting the Right Product for Your Needs
Choosing a financial product requires careful consideration of your investment goals, risk tolerance, and timeline. Factors to ponder:
- Time Horizon: Are you looking for short-term gains or long-term stability?
- Risk Tolerance: Will you weather fluctuations in the market, or do you prefer safety?
- Research: Always exercise due diligence—understand the intricacies of each product before jumping in.
Getting these aspects right enhances your modelling ability and overall confidence in financial dealings.
"The best investment you can make is in yourself. The more you learn, the more you earn." – Warren Buffett
Understanding these foundations sets the stage for deeper dives into various modelling techniques and the tools that make them possible, two areas we’ll explore as we progress through this guide.
Understanding Financial Modelling
Financial modelling serves as the bedrock of decision-making in the fast-paced and often turbulent world of Wall Street. It's not merely about crunching numbers; it’s about telling a story through data. The importance of understanding financial modelling cannot be overstated. Its strategic role enhances investment analysis, risk management, and performance forecasting. By employing comprehensive models, analysts can project earnings, assess investment viability, and guide corporations in making informed financial choices.
Definition and Importance
At its core, financial modelling involves creating a mathematical representation of a financial scenario. This entails using historical data combined with assumptions to forecast future financial performance. The models may encompass a variety of tools, from simple spreadsheets to intricate software systems. This nuanced understanding is key because the financial landscape is treacherous—judicious decisions can lead to thriving businesses, while miscalculations often result in substantial losses.
The primary benefits of financial modelling include:
- Investment Evaluation: Models facilitate the analysis of potential returns relative to risks, ensuring funds are allocated efficiently.
- Predictive Insights: Forecasts generated from models help in anticipating market changes, giving firms a leg up on their competitors.
- Strategic Planning: Organizations use these models to create budgets and operational plans that align with their strategic goals.
In summary, grasping the intricacies of financial modelling equips investors, financial advisors, and analysts with the requisite tools for effective decision-making. It separates the wheat from the chaff in an industry where precision dictates survival.
Historical Context
To truly understand financial modelling, one must look back at its evolution. Financial modelling isn't a modern phenomenon; it has roots tracing back to the early 20th century. Initially, models were rudimentary, often limited to simple calculations of cash flows and basic projections. However, as markets grew and technology advanced, so did the complexity and sophistication of these models.
In the 1970s and 1980s, the advent of computers revolutionized the field. Analysts began employing software like Lotus 1-2-3 or Microsoft Excel, allowing for more complex and iterative models. This evolution made real-time adjustments feasible, enhancing responsiveness to changing market conditions.
Moreover, major financial crises in history have highlighted the necessity for robust financial modelling. Events like the 2008 financial crisis underscored the importance of deep, layered analysis. It became clear that inadequate modelling could lead to catastrophic miscalculations.
Thus, the history of financial modelling illustrates not just its growth, but also its ongoing relevance in shaping modern financial strategies. To navigate today’s fickle markets, understanding this context provides a significant advantage.
Key Components of Financial Modelling
When navigating the world of Wall Street, understanding the key components of financial modelling becomes essential. These components act as building blocks in constructing sound financial analyses that help investors make informed decisions. Each element plays its part in creating a comprehensive picture of a company's financial health and forecasts. Let's peel back the layers and examine these important facets in greater detail.
Common Financial Models Used on Wall Street
In the bustling world of Wall Street, financial models are crucial tools that guide investment decisions and strategic financial planning. These models allow analysts and investors to forecast revenues, assess risks, and value companies. Understanding these common financial models can empower financial professionals to make informed decisions and mitigate potential missteps. As such, knowledge of these frameworks is not just beneficial; it’s paramount for navigating the complexities of the financial landscape.
Discounted Cash Flow (DCF) Model
The Discounted Cash Flow (DCF) model stands as one of the cornerstones of financial analysis. At its core, this model helps in determining the value of an investment based on its expected future cash flows. It operates on the principle that money available today is worth more than the same amount in the future due to its potential earning capacity. Therefore, the DCF model discounts these future cash flows back to their present value using a discount rate, which usually reflects the investment’s risk profile.
The DCF approach is particularly well suited for investments that generate clear and predictable cash flows, such as companies in stable industries. However, it has its pitfalls. The accuracy of DCF is heavily reliant on the inputs used, specifically cash flow projections and the discount rate. Both elements can be subject to bias and inaccuracies. To maximize its value, analysts must not only rely on quantitative data but also consider qualitative factors like market trends and industry shifts.
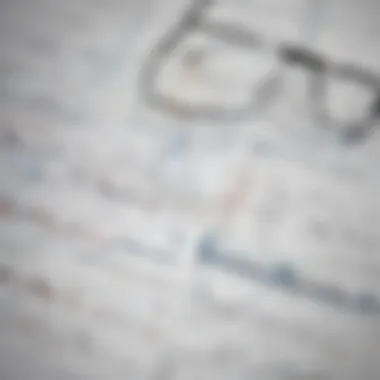
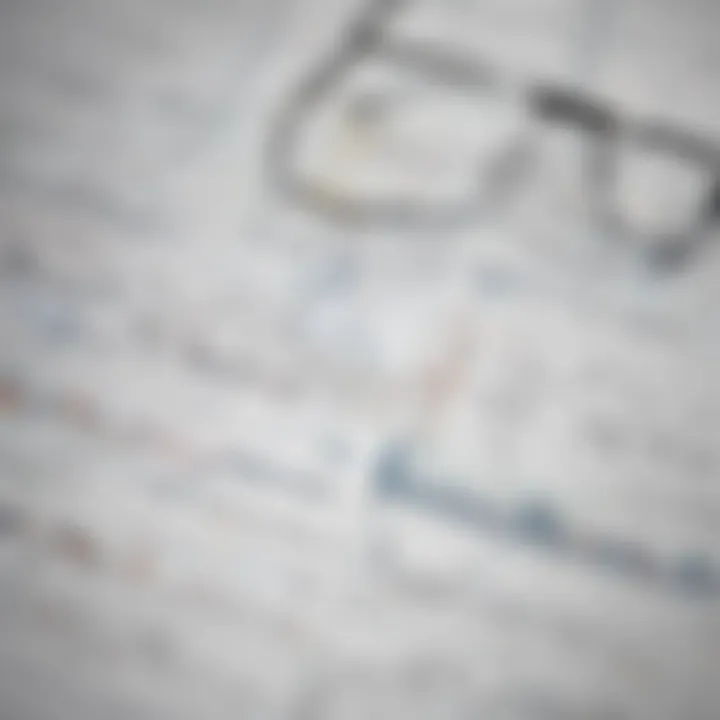
Comparable Company Analysis
Another widely used valuation technique is Comparable Company Analysis (Comps). This method assesses the value of a company by comparing it to similar businesses within the same industry. By looking at metrics such as Price-to-Earnings (P/E) and Enterprise Value-to-EBITDA ratios, investors can gauge how the subject company stands relative to its peers.
Using Comps offers a real-time market perspective, as it takes into consideration how the market values different companies at a given time. However, it requires a careful selection of comparable companies, which need to share similar traits, such as market size, growth rates, and operational structure. The reliability of this model diminishes if the comparison is not apt, leading to potential overvaluations or undervaluations.
Precedent Transactions Analysis
Precedent Transactions Analysis, often referred to as M&A Comps, provides insight into how much similar companies have been bought or sold for in the past. The approach involves analyzing historical transactions within the same industry to establish a range of valuation multiples.
This method proves valuable in acquisition scenarios, allowing a buyer to see what prices have been accepted for similar assets. However, it’s worth noting that market conditions, negotiation tactics, and the strategic fit of a company can skew results. Thus, the context of each transaction must be evaluated critically to glean meaningful insights.
LBO Models
Leveraged Buyout (LBO) models are utilized mainly by private equity firms to assess potential acquisitions using significant amounts of borrowed money. These models project the cash flows of the company post-acquisition and determine how much debt the firm can service. A key aspect of LBO modeling is understanding the leverage ratio, which reflects how much debt is being used to finance the purchase compared to the equity involved.
One of the main advantages of an LBO is the opportunity for high returns. When the investment succeeds, the substantial debt can amplify profits. Nonetheless, the model also emphasizes risk; if the company fails to generate sufficient cash flows, servicing the debt can become untenable. The model illustrates the delicate balancing act required in leveraged finance, making it essential for investors to conduct thorough due diligence before proceeding.
"Understanding these models provides a lens through which investors can navigate the complex waters of financial valuation, risking neither efficiency nor accuracy."
Building a Financial Model
Constructing a financial model is a cornerstone of economic analysis on Wall Street. It serves not merely as a tool for projecting future revenues and expenses, but as a lens through which investors can peer into the potential financial outcomes of their decisions. A well-structured financial model can unravel complex scenarios, allowing users to navigate the often-turbulent waters of financial markets.
When building a model, the importance lies not just in omitting errors, but in creating a flexible framework that can adapt to changing variables. Investors and analysts depend on these models for insights that guide their investment strategies and risk assessments. This flexibility can make the difference between spotting a lucrative opportunity and missing the boat altogether.
Outline of the Process
Building a financial model typically follows a methodical approach, ensuring that all relevant factors are captured accurately. The general outline often comprises:
- Define the Objective: Knowing the goal of the model is paramount.
- Gather Data: Collect historical data and define assumptions.
- Structure the Model: Create a logical flow that includes key components like revenues, expenses, and cash flow.
- Develop Scenarios: Account for best-case, worst-case, and expected outcomes.
- Review and Revise: Continuous iteration based on feedback ensures reliability and accuracy.
Each of these steps plays a vital role in guaranteeing the model's effectiveness. A hasty or poorly thought-out approach during any phase can lead to miscalculations that may prove costly down the line.
Choosing the Right Software
Selecting the appropriate software for financial modelling is akin to choosing a sturdy boat for a long voyage. Excel remains the unanimous favorite among professionals due to its flexibility and power. However, there are specialized tools like Quantrix, Anaplan, and Adaptive Insights that can provide additional functionalities tailored to intricate modelling tasks. Such software can help streamline data integration and offer enhanced visualization options, making the modelling process more intuitive.
The choice of software should align with the complexity of the model and the end-user’s proficiency. Also, considering collaborative features could facilitate teamwork, especially in large organizations.
Data Collection and Input
Data serves as the foundation of any financial model. Collecting accurate and comprehensive inputs is not a one-size-fits-all scenario. Investors must:
- Access Reliable Sources: Financial statements, market research, and economic indicators should come from trusted providers.
- Adjust Historical Data: The past isn't a crystal ball. Adjust for one-time events to get a clearer picture of the ongoing trends.
- Input Constantly Updated Figures: Financial dynamics change swiftly; ensuring your figures reflect current market conditions is essential for accurate projections.
Proper data collection isn't merely about filling cells in a spreadsheet; it is about ensuring every figure is an accurate representation of the envisioned financial outcomes.
Scenario Analysis
Engaging in scenario analysis adds an extra layer of robustness to your financial model. By simulating different market conditions or operational changes, you can identify how your financial outcomes might shift under varying circumstances. This includes assessing:
- Best-Case Scenarios: What if everything goes right?
- Worst-Case Scenarios: How will the model respond to significant downturns?
- Most Likely Outcomes: A grounded expectation based on current trends.
Scenario analysis isn’t about predicting the future; it’s about preparing for a range of possibilities. Following these assessments, you should incorporate the findings back into the model, adjusting assumptions where needed.
"A financial model is not a static entity but a living document that evolves with every insight and piece of data we gather."
Employing sound practices in financial modelling can truly serve to illuminate opportunities that might otherwise remain concealed. A deep understanding of the entire process—from outlining your model to scenario testing—can elevate one's decision-making capabilities and lead towards informed financial strategies.
Advanced Modelling Techniques
Advanced modelling techniques play a pivotal role in the realm of financial modelling. They serve as crucial tools that aid professionals in analyzing data with greater depth. These methods go beyond simple projections, offering insights that can inform better business and investment decisions. Each technique brings its own benefits, but a common thread is the ability to increase the accuracy of financial forecasts.
An essential aspect of advanced modelling is the ability to handle complexity. Financial landscapes are often fraught with uncertainties; therefore, models need to adapt to the changing environment. Using advanced techniques allows modellers to account for various scenarios, making them a fundamental part of an analyst’s toolkit. Now, let’s delve into some key advanced modelling techniques.
Monte Carlo Simulations
Monte Carlo simulations are a powerful method for assessing risk and uncertainty in financial forecasts. This technique uses random sampling and statistical modeling to understand the impact of risk and uncertainty in prediction and forecasting models. By simulating a range of possible outcomes, it provides a distribution of potential financial scenarios.
For instance, imagine a company looking at its investment in a new product line, considering multiple variables including cost of goods, market demand, and marketing expenses. Through Monte Carlo simulations, analysts can project a range of possible revenues, highlighting not just the most likely outcome but also best and worst-case scenarios. This allows decision-makers to make informed choices backed by quantitative data.
"Monte Carlo simulations turn uncertainty into a tool for clarity in decision-making."
This technique reflects the real world more accurately than deterministic models, which only provide a single outcome. Companies employing Monte Carlo methods gain a more nuanced view of their risk landscape, enabling them to devise strategies that minimize potential downsides.
Sensitivity Analysis
Sensitivity analysis is another advanced modelling technique that assesses how sensitive a model’s outcomes are to changes in input variables. It sheds light on which assumptions or variables have the most influence on results.
For example, a financial modeller might evaluate how fluctuations in interest rates or market demand affect a company's projected earnings. By varying these inputs and observing changes in output, analysts can pinpoint critical thresholds. This can be visualized succinctly through sensitivity charts, where inputs are plotted against outputs, providing a clear picture of risk and opportunity.
Conducting effective sensitivity analysis can lead to better strategic planning. Analysts can focus efforts on variables that significantly impact outcomes, enhancing both the precision of forecasts and the robustness of strategies derived from them.
Time-Series Forecasting
Time-series forecasting is a statistical technique used to predict future values based on previously observed values. This technique is especially useful in finance as markets often follow patterns over time. Analysts can utilize historical data to project future performance with a fair degree of accuracy.
Consider a financial analyst examining historical stock prices. By applying time-series analysis, they can identify patterns or trends — seasonal variations, recurring cycles and other time-dependent behaviors. This provides insights into the likely future performance of a stock, enabling investors to make informed decisions.
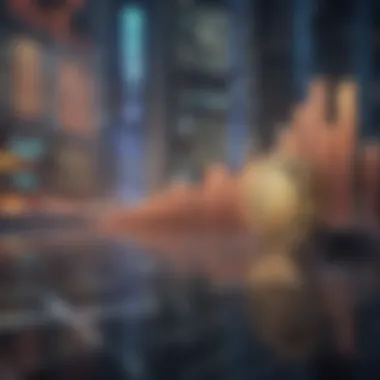
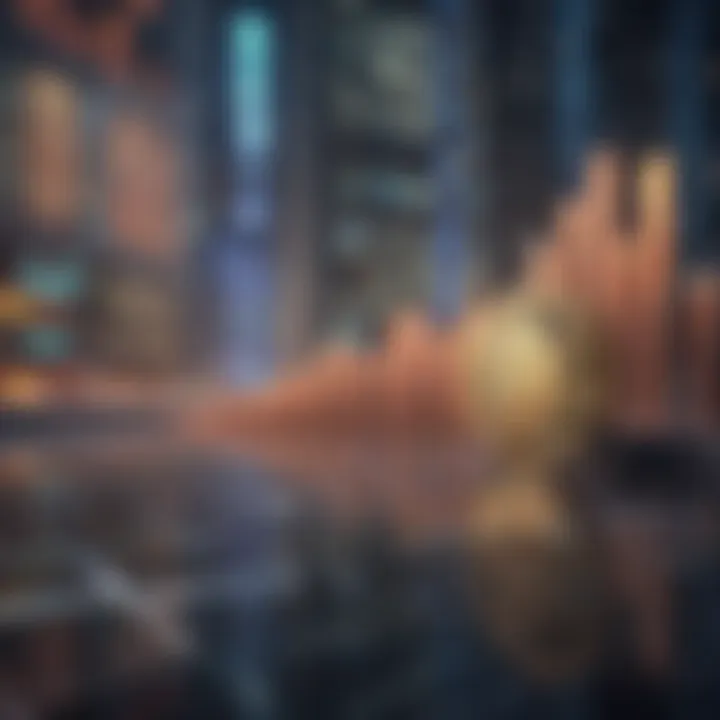
Models such as ARIMA (AutoRegressive Integrated Moving Average) and exponential smoothing techniques can be employed for more rigorous time-series analysis. These methods help refine predictions, increasing their reliability and utility in decision-making for investors and financial advisors alike.
In summary, the use of advanced modelling techniques such as Monte Carlo simulations, sensitivity analysis, and time-series forecasting enhances the accuracy and robustness of financial models. They allow financial professionals to navigate complexities and uncertainties effectively, leading to more informed strategic decisions that align with the dynamic nature of the markets.
Risk Assessment in Financial Modelling
Risk assessment plays an integral role in financial modelling, particularly within the high-pressure atmosphere of Wall Street. Assessing risks involves identifying potential pitfalls that could derail a financial projection or strategy. It’s not just about crunching numbers or interpreting market trends; it’s about understanding the uncertainties that can impact the credibility and effectiveness of the model being used.
By systematically identifying risks, evaluating their impact, and developing mitigation strategies, financial professionals create more robust models. The key benefits of effective risk assessment are many:
- Improved decision-making quality
- Enhanced credibility with stakeholders
- Increased adaptability to market changes
Factors to consider include the dynamic nature of financial markets, the influence of global events, and the sensitivity of models to various assumptions. In short, understanding risk is like having a crystal ball; it brings clarity to the murky waters of financial forecasting.
Identifying Risks
The first step in risk assessment is identifying the specific risks that could affect the financial model. These risks can be diverse, ranging from market volatility to operational hiccups or even unforeseen regulatory changes. It’s essential to look at both internal and external factors that could cause discrepancies in the model's projections.
Common categories of risks include:
- Market Risk: Fluctuations in market prices and interest rates.
- Credit Risk: The potential for default by borrowers.
- Liquidity Risk: Inability to convert assets into cash quickly without loss.
- Operational Risk: Failures in processes, systems, or human resources.
Identifying these risks often involves brainstorming sessions and consultations with various departments. It’s also prudent to utilize historical data to pinpoint previously encountered risks and consider their consequences.
Evaluating Impact
Once risks have been identified, evaluating their potential impact comes next. This step requires a keen analytical eye, as not all risks carry the same weight. Some may have minimal consequences, while others could have catastrophic effects on the model and, by extension, the firm’s financial health.
Techniques to assess impact may involve:
- Quantitative Analysis: Calculating the likelihood of the risk and estimating the financial loss in case it materializes.
- Scenario Analysis: Constructing various scenarios (best-case, worst-case, and medium-case) to understand how each risk could unfold.
- Stress Testing: Running the model under extreme conditions to gauge its resilience.
This incorporation of structured impact assessment equips financial advisors and investors alike to prepare for various eventualities, ensuring that they are not caught off guard by market movements.
Mitigation Strategies
After evaluating the impact of identified risks, the next logical step is to devise mitigation strategies. Having a plan in place allows for a more agile response if and when those risks materialize. The goal here is not to eliminate risks entirely—after all, in the world of finance, some degree of risk is inherent—but to manage them effectively.
Potential strategies include:
- Diversification: Spreading investments across different asset classes to minimize risk.
- Hedging: Using financial instruments such as options or futures to offset potential losses.
- Regular Model Updates: Keeping the model current with the latest data and trends ensures relevant assumptions are maintained.
- Contingency Plans: Establishing procedures for rapid response in case a risk event occurs, ensuring minimal disruption.
Thus, managing risks is not merely an add-on to financial modelling; it’s an essential practice that, when implemented well, naively enhances the model’s reliability and the overall strategic decision-making process.
Ethics and Compliance in Financial Modelling
Financial modelling isn't just a matter of crunching numbers and projecting future returns; it embodies a set of ethical principles and compliance standards that govern the behavior of financial professionals. In the high-stakes arena of Wall Street, where money talks and decisions ripple across markets, having a robust ethical framework isn't optional—it's imperative. A focus on ethics and compliance not only fosters trust among investors but also enhances the credibility of the modelling process itself.
Regulatory Frameworks
The landscape of financial regulation is as convoluted as a labyrinth, with multiple layers to navigate. Regulatory frameworks such as the Sarbanes-Oxley Act and the Dodd-Frank Act, for instance, set strong guidelines in place regarding financial reporting and corporate governance. These laws serve as a check against fraud, ensuring that financial models are built on a solid foundation of truthfulness and integrity.
From the SEC’s rigorous oversight to stringent workplace compliance programs, financial institutions must adhere strictly to these regulations. Non-compliance can lead to hefty fines, reputational damage, or worse, legal consequences. It paints a very clear picture that the adherence to these regulations is not just about avoiding penalties; it's about creating a trustworthy environment for all parties involved.
Best Practices
Establishing a firm ethical footing is not just about regulatory compliance; it's about fostering a culture of integrity across all financial practices. Here are some best practices that can help achieve that:
- Transparency: Always disclose methodologies, data sources, and assumptions used in creating financial models. This prevents misinterpretation and enhances clarity.
- Regular Audits: Conduct regular internal audits of financial models to ensure compliance with established standards and to identify possible ethical breaches.
- Continuous Training: Offer ongoing training to staff about the latest regulations and ethical standards in financial modelling. Knowledge is power, and well-informed employees are less likely to step off the ethical path.
- Whistleblower Protection: Implement a system that protects whistleblowers. Employees should feel safe reporting unethical behavior without fear of repercussions.
Being rigorous about ethics and compliance ensures that financial models serve their intended purpose: guiding decisions based on reliable, truthful information. Maintaining a commitment to these values not only protects an institution's reputation but also contributes to a more stable and trustworthy financial marketplace.
"Ethics is not a burden; it’s the foundation of a functioning financial system."
Technological Innovations Shaping Financial Modelling
In today's financial landscape, technology plays an indispensable role in shaping how financial modelling is crafted and implemented. With the fast-paced environment of Wall Street, professionals are leaning more heavily on technological innovations to enhance accuracy, provide deeper insights, and improve overall efficiency. Understanding these technologies helps investors, financial advisors, and analysts navigate the complex waters of investment strategies and risk management.
Artificial Intelligence and Machine Learning
Artificial intelligence (AI) and machine learning (ML) are not just buzzwords; they're evolving into crucial components of financial modelling. These technologies allow analysts to automate data analysis and predict future trends more reliably. By leveraging vast amounts of historical data, AI algorithms can uncover patterns that might elude human analysts.
For example, an AI model can process thousands of financial transactions and news articles at lightning speed, identifying potential risks long before they become evident to a human eye. Moreover, machine learning can refine its predictions as more data becomes available, which brings a level of adaptability unheard of in traditional modelling methods.
Additionally, AI can assist in sentiment analysis. Imagine a model that analyzes Twitter feeds and news headlines to gauge market sentiment about a specific company or sector. Such tools provide financial professionals with a broader understanding of market dynamics, thus aiding in more informed decision-making.
Blockchain Technology
Blockchain technology is here, and it’s upheaving traditional practices in almost every sector, finance included. Its decentralized nature means that data is not owned by one entity but distributed across a network. This peer-to-peer infrastructure enhances transparency and traceability, which are vital elements for any financial model.
For instance, blockchain can secure financial transactions and improve traceability in asset movements. This aspect minimizes fraud risk and allows for the real-time updating of financial models based on verified data. When financial modelling incorporates blockchain, not only is accuracy boosted, but operational costs are often cut in half due to the reduced need for intermediaries.
Experts predict that as blockchain matures, it could revolutionize how data is shared and modeled within financial services. However, the volatility associated with blockchain assets is something investors need to keep an eye on, as it can dramatically influence models relying on those assets.
Cloud Computing Solutions
The move to cloud computing has transformed the way financial modelling is approached. Many firms are making the shift from traditional on-premise infrastructures to cloud-based solutions, a change fueled by the need for scalable computing resources. The cloud enhances collaboration among teams, regardless of their physical locations. This provides a significant advantage, especially for firms with inter-departmental teams working on extensive modelling projects.
Not only can resources be allocated much more efficiently, but cloud platforms also support advanced analytics tools that can process complex models at high speeds. Moreover, they often come with built-in security protocols, making them more secure than many in-house systems.
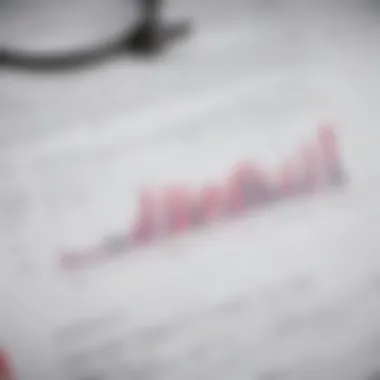
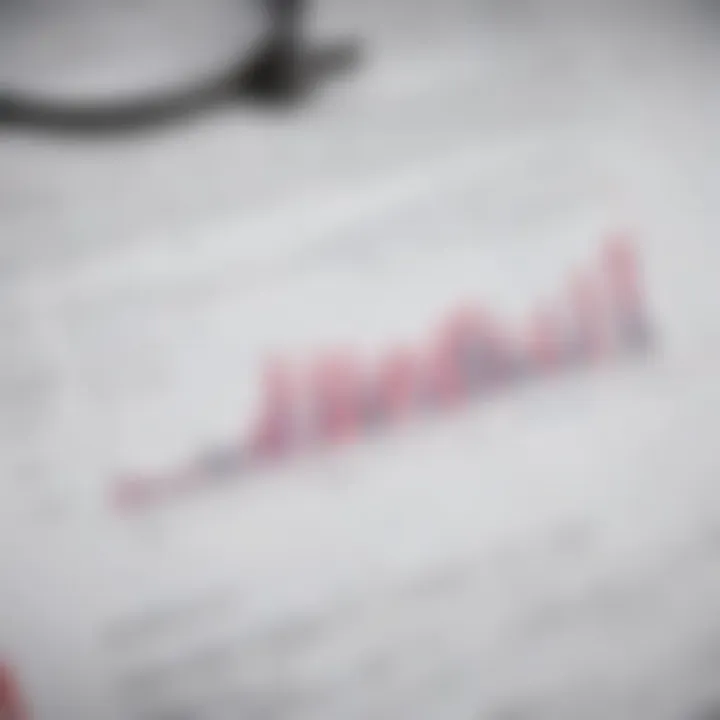
In short, cloud technology allows financial professionals to access powerful modelling tools without needing extensive infrastructure investments. As firms continue to leverage these solutions, it’s likely that cloud computing will underpin many future financial modelling processes.
The integration of technology in financial modelling is set to redefine what analysts can achieve, pushing the boundaries of accuracy and efficiency.
Assessing the Validity of Financial Models
In the complex world of finance, the validity of financial models is not just a theoretical exercise; it's pivotal for investment decisions, risk management, and corporate strategy. As various methods and tools are employed to forecast economic outcomes, it becomes essential to ensure that these models are grounded in accuracy and reflect real-world dynamics. Failing to assess validity can lead to misguided decisions, wasted resources, and, in some instances, significant financial losses. Let's delve into the key elements of how the validity of financial models can be evaluated, highlighting the importance of rigorous testing and peer review.
Model Testing and Back-Testing
Model testing refers to the process of evaluating the performance of a financial model against historical data to gauge its predictive power and reliability. This involves comparing the outcomes yielded by the model with actual results observed in the market. There are a few essential techniques used:
- Out-of-sample Testing: By using data not included in the model's construction phase, analysts can assess how the model performs under different market conditions. This step is crucial for validating the robustness of the model.
- Stress Testing: This involves subjecting the financial model to extreme market conditions—such as a market crash or a sudden spike in interest rates—to see how well it holds up. It helps in identifying potential weaknesses.
- Error Calculation: Analysts often use metrics like Mean Absolute Error (MAE) or Root Mean Squared Error (RMSE) to quantify how far off the model's projections are from actual outcomes. Keeping track of these errors can provide insight into needed adjustments.
Back-testing enhances the credibility of a model by using it to make predictions from past data and then comparing those predictions to what actually happened. A model that consistently predicts results accurately during back-testing is deemed more reliable. However, it’s critical to note that past performance does not guarantee future results; thus, models should be constantly refined.
"A model that’s proven during turbulent times holds water in calm seas, but the reverse can be just as misleading."
Peer Review Process
The peer review process serves as a vital checkpoint in assessing the validity of financial models. Here, the work of analysts is subjected to scrutiny by other experts in the field. This collaborative approach has several advantages:
- Objective Feedback: Input from peers can help uncover potential flaws, biases, or assumptions that the model creator may overlook. Fresh eyes can spot mistakes or suggest alternative approaches that enhance the model's effectiveness.
- Standardization: During peer review, financial models can be aligned with industry standards. This ensures that the model is grounded in sound financial theory and components recognized in the marketplace.
- Transparency: A model that has undergone a thorough peer review process tends to instill more faith in its users. Financial stakeholders can feel more comfortable relying on it for decision-making, knowing that it has been vetted by experts.
Case Studies in Wall Street Financial Modelling
Understanding financial modelling on Wall Street is not just about the numbers in spreadsheets or the algorithms running behind the scenes; it’s about seeing the practical implications of those models in real-world scenarios. This segment closely examines both the triumphs and shortcomings of financial models through various case studies. By analyzing these case studies, we gain insights that are instrumental for investors, financial advisors, and analysts aiming to refine their own modelling practices.
Here are some key points on the importance of case studies:
- Real-World Applications: Case studies offer concrete examples of how financial models perform in practice, providing a reality check against theory.
- Learning from Experience: They highlight lessons learned from both successful and failed financial models, allowing others to avoid similar pitfalls.
- Evolving Standards: They showcase how financial models adapt over time to changing market conditions and regulatory environments.
- Gaining Perspective: Analyzing both the wins and losses within different contexts enables a more holistic view of financial modelling as a discipline.
"In the world of financial modelling, every number tells a story, and every story holds a lesson."
Successful Models: Lessons Learned
One shining example of a successful financial model comes from the tech sector, particularly the rise of Netflix. When it first ventured into original content, Netflix utilized a robust DCF model that projected future earnings based on subscriber growth and content spending. Analysts bespoke intricate assumptions, ensuring to capture various growth scenarios and competitive pressures. This model provided stakeholders with a clearer picture of the company’s trajectory, solidifying investor confidence.
Lessons Learned
- Emphasize Assumptions: Strong assumptions led to more reliable projections, demonstrating the need for depth in underlying data.
- Monitor Changes: Continuous updates based on market changes helped Netflix stay ahead of competitors, showcasing the importance of adaptability in financial modelling.
- Communicate Effectively: Netflix’s management communicated the rationale behind their projections transparently, building trust with investors.
In essence, the success of Netflix's model lay not just in the accuracy of its predictions, but also in how well they integrated feedback and tweaked their assumptions in real-time.
Failures: What Went Wrong
Contrastingly, let’s look over at Enron, a name that has become synonymous with corporate deceit and failed financial modelling. Enron’s financial model appeared to be groundbreaking, yet it completely collapsed due to its reliance on overly optimistic revenue forecasts and hidden liabilities.
Key Takeaways from Failures
- Overly Complex Models: Enron developed intricate financial structures, which obscured reality rather than clarified it. The lesson here is that simplicity can often be more effective than complexity.
- Lack of Transparency: They failed to communicate effectively with investors about the real state of their finances. Clear, honest communication is vital for a successful model.
- Ignoring Risks: Enron's disregard for potential risks—with blind faith in their own projections—exemplifies the perils of not assessing risks sufficiently.
In studying these case studies, practitioners can draw lessons that are not only relevant but critical for their own modelling endeavours. These insights underscore the fact that both successes and failures contribute to the ever-evolving landscape of financial modelling on Wall Street.
Future Trends in Financial Modelling
In the ever-evolving landscape of financial modelling, recognizing future trends is instrumental for both seasoned investors and newcomers. The adaptability of financial models to shifting market dynamics underpins their long-term relevance and effectiveness. Anticipating these trends not only sharpens competitive edges but also aligns strategies with market needs. As technology permeates finance, understanding these future directions becomes paramount.
Emerging Methodologies
With the rise of sophisticated analytics and data availability, emerging methodologies are transforming financial modelling practices. Here’s a closer look at some prominent trends:
- Agile Financial Modelling: Traditional models often suffer from rigidity. Agile methodologies allow for more adaptable forecasting and quicker adjustments to inputs like market conditions or regulations. This approach enables firms to pivot faster when surprises come up.
- Holistic Modelling Approaches: Instead of standalone models, a more interconnected view is emerging. This involves combining various models to reflect broader market sentiments or multiple business elements, giving a well-rounded perspective on potential outcomes.
- Custom Algorithms: The role of machine learning in financial modelling is gaining traction. Financial professionals now can design tailored algorithms that analyze data from diverse sources – from market trends to social media sentiment. This can lead to insights that traditional methods miss.
- Visual Modelling Techniques: Technology isn’t just about data analysis; it also involves representation. Visual modelling techniques help convey complex information more digestibly, aiding decision-makers around the table.
Adopting these methodologies means acknowledging the intricacies of financial data while pushing towards simplification, helping to ensure better comprehension across all levels of understanding within organizations.
Changing Market Dynamics
Market conditions are far from static, and as they shift, financial modelling needs to reflect these changes sustainably. Some key transformations include:
- Increased Volatility: The post-pandemic market has brought heightened volatility. Financial models must now account for rapid fluctuations due to economic events or geopolitical tensions. This requires embrace of scenario planning and stress tests that highlight resilience in varied conditions.
- Regulatory Evolution: Constant changes in regulations impact the financial landscape. Models must stay current with these changes, ensuring compliance while still providing reliable forecasts. Firms that stay ahead of regulatory changes can capitalize on new opportunities and minimize risks.
- Sustainability Focus: Investors are increasingly inclined towards sustainable investments. Financial modelling must evolve to incorporate environmental, social, and governance (ESG) factors into assessments. This trend not only reflects a changing investor psyche but brings new dimensions to financial evaluation.
"Adaptability isn't just an asset; it’s a necessity for survival in a tumultuous market."
Finale
The journey through the landscape of Wall Street financial modelling reveals much more than just numbers and graphs; it highlights a cornerstone of decision-making in the financial sector. Through our exploration, we understand that financial models serve vital purposes, from forecasting future performance to conducting thorough analyses of investment opportunities. The accuracy of these models significantly impacts strategic choices, influencing actions that can lead to substantial financial gains or losses.
Key elements such as assumptions, data integrity, and adaptability to changing market conditions emerge as fundamental considerations when utilizing these models. Successful navigation through the financial landscape hinges on solid models that account for various scenarios, helping investors and analysts alike to make informed decisions.
Recap of Key Insights
In our detailed examination, we noted that:
- Diverse Models: Various financial models like DCF, comparable company analysis, and precedent transactions have unique applications tailored to specific situations in investment decision-making.
- Importance of Software: The right software tools can enhance the modelling process, facilitating data collection, analysis, and presentation.
- Risk Management: Understanding and mitigating risks is crucial; otherwise, financial projections may spiral into inaccuracies.
"In finance, what you see on the surface is merely a fraction of its depth, revealing the critical role of sound modelling practices in risk assessment and opportunity identification."
These insights underscore the intricate relationship between financial modelling and strategic financial management on Wall Street.
The Road Ahead for Financial Modelling
As we look forward, several trends are emerging that will shape the future of financial modelling:
- Enhanced Technologies: The integration of machine learning and artificial intelligence into financial modelling is expected to drive accuracy and efficiency. Investors will increasingly rely on these innovations to interpret vast amounts of data.
- Real-Time Data Utilization: Vast advancements in cloud computing will allow real-time data collection and processing, enabling quicker reactions to market changes.
- Increased Regulation: Stricter regulatory frameworks demand transparency and accuracy, pushing financial professionals to continuously refine their models to meet compliance standards.